A Machine Learning approach to material classification in Additive Manufacturing
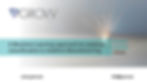
Abstract
The objective of this work was to develop a machine learning (ML) system for automatic identification of the filament type used by Fused Deposition Modelling (FDM) 3D printers. This system, in conjunction with other assessments, could be used in additive manufacturing (AM) as a monitoring and quality control tool during 3D printing process. The major advantage of this approach is that monitoring of the printing process can be performed in a simple, completely non-invasive way, without having any additional impact on the printing process. This system can be implemented for real-time control monitoring on the layer-basis by independently processing data acquired for a single layer and, as a result, issuing a warning if type of the filament has been changed during printing process.
In this report, a methodology developed with aim to recognise usage of different filament type than the one predefined in the parameter settings during printing of a sample design is presented. The methodology consists of a set of techniques employed to acquire and process the sensor data, compute a set of specific task-related features and classify data according to the filament type used. In order to prove the concept, an experiment was designed and performed on the FDM printer to collect data for analysis. Preliminary test results of the proposed and implemented ML system evaluated from the experimental data demonstrates the detection rates of 82-97%. The achieved results represent the initial steps in development of an automated real-time system for controlling filament type in the FDM systems.
To learn more, contact us at info@grow.am.
Download the complete white paper from: A Machine Learning approach to material classification in Additive Manufacturing